Table of Contents
Predictive Analytics goes hand in hand with Artificial Intelligence. Both require large amounts of data, but they work very differently. While AI is autonomous and adapts, predictive analytics relies on historical data and human intervention to forecast future events.
Generative AI vs. predictive AI also accounts for this same difference, where generative AI models create new content, while predictive AI simply forecasts. Predictive analytics vs. artificial intelligence is about the scope of learning in AI, how it adapts, and how it performs complex tasks, while predictive analytics focuses on trend identification.
This article is about the AI vs predictive analytics comparison, where you will learn 10 significant differences between them. From business uses to data requirements, this is a comprehensive guide to AI vs. predictive analytics for you.
What is Artificial Intelligence?
Artificial Intelligence, or AI, is a system that can perform tasks that would otherwise require human intelligence, such as learning, reasoning, and problem-solving.
AI is a continuously improving system that learns from its mistakes, past behaviors, and adjusts its model, making it adaptable and autonomous. A few famous applications of AI include machine learning, deep learning, neural networks, and others.
Businesses today are seeking data-driven insights to be competitive. 2 major approaches are AI and predictive analytics. Both work well for the companies’ needs. But when it comes to comparing AI and predictive analytics for business, knowing the differences helps.
For strategic decision-making, AI and predictive analytics tools help simplify complex tasks, choosing between AI and predictive analytics for business a significant decision in itself.
What is Predictive Analytics?
Predictive analytics is the combination of statistical techniques and machine learning algorithms to analyze historical data and forecast future outcomes. Unlike AI, which needs less human input, predictive analytics requires much more. Things like selecting variables and validating model assumptions require human intelligence and experience.
The main goal is to find patterns in behavior trends, risks, or other variables and then predict future outcomes, combining statistics with machine learning to support data-driven decisions.
AI vs Predictive Analytics: 10 Key Differences
1. Autonomy vs. Human-Guided
AI systems follow what is called unsupervised learning, which makes them autonomous. They learn, adapt, and update models as new data is fed to the Model. AI models don’t need constant supervision. Predictive Analytics, on the other hand, depends heavily on analysts or human supervision. Humans are needed for tasks such as identifying the problem, selecting data, interpreting the results, and more.
2. Scope of Application
AI’s applications span a broad array of tasks, including image recognition, speech recognition, text analysis, language translation, robotics, and more. Predictive analytics focuses on finding trends that can be used in sales forecasts, risk assessments, and similar tasks.
3. Generative AI vs Predictive AI Models
Generative AI models create new content from existing data, such as images, text, and music, using Generative Adversarial Networks (GANs) and Large Language Models (LLMs). Predictive Analytics forecasts outcomes such as sales, customer retention, and demand. They need elementary algorithms and much smaller datasets.
4. Data Requirements
AI, especially generative AI, requires massive and diverse datasets for training to ensure adequate training and minimize biases as much as possible. Millions of text samples and images are needed for the average dataset. Predictive analytics can work efficiently with more targeted, smaller datasets, which are primarily focused on predicting variables relevant to forecasting.
5. Model Complexity
AI models are very complex, with multiple layers and parameters, especially those in deep learning. Predictive analytics, on the other hand, uses much simpler static models, such as ML techniques like regression and decision trees.
6. Interpretability
Predictive Analytics is easily interpretable on its own, where inputs drive outputs. AI models often need another technique to interpret their results, such as the SHAP value. Intense neural networks will need it.
7. Speed
AI models and systems can easily process and learn from live streaming data in real-time, and adjust recommendations instantly as well. Predictive analytics will operate in batches. Analysts run forecasts periodically based on the collected data sets.
8. AI vs Predictive Analytics Explained: Use Cases
AI has multiple use cases in autonomous vehicles, chatbots, image generation, adaptive learning, and more. Predictive analytics is in demand for forecasting, credit scoring, scheduling, marketing optimization, and weather forecasting, among others. Both have reasonable demands in their respective areas of application.
9. Cost and Infrastructure
AI requires ample computing resources because it needs to work on millions of data units. Generally, specialized massive GPUs, such as TPUs, are needed along with data engineering and frameworks like TensorFlow and PyTorch. This means a very high cost of setup and infrastructure. Predictive analytics is cheaper and can be run efficiently on standard CPUs and GPU devices, using lightweight tools like scikit-learn.
10. Evolving Roles
AI and predictive analytics both have their respective roles in business and in general. Data science combines AI and predictive analytics tools to build models, interpret results, and gain insights.
But AI is primarily used for roles that require massive data, quick yet complex computing, and repetitive tasks. Predictive analytics is used for roles such as forecasting and finding trends, anywhere it may be applicable. AI is even used to generate data to train predictive analytics models.
Tools and Frameworks
- AI frameworks: such as TensorFlow and PyTorch are used for building generative and deep learning models.
- Predictive analytics tools: such as SAS Predictive Analytics, IBM SPSS, Python’s scikit-learn, and RapidMiner, are traditionally used for predictive analytics.
- Combined solutions: There are combined solutions such as Azure Machine Learning, Google Cloud API Platform, and AWS Sagemaker. These support both AI and predictive analytics work and tasks to simplify the AI vs predictive analytics for business implementation.
Business Applications of AI and Predictive Analytics in Various Fields
1. Marketing Optimization
AI is used for personalized content generation, such as chatbots, and predictive analytics are used for customer forecasts and calculating campaign return on investment (ROI).
2. Healthcare
AI helps in analysing images, X-rays, CT scans, etc, for diagnostics. Predictive analytics models will help identify patient readmission rates, risk, and treatment outcomes based on the diagnostic results received.
3. Finance
AI monitors transactions in real-time to detect fraud patterns, while predictive analytics helps analyze credit scores and credit risks.
Conclusion
AI and predictive analytics each have their business potential. Predictive analytics vs. artificial intelligence helps a business make more informed decisions and approach its specific problems. While AI does have its broad autonomous abilities, predictive analytics helps find more targeted forecasts, allowing businesses to prepare in advance.
This is where experienced technology partners like Shiv Technolabs come in, offering tailored AI and data analytics solutions to help organizations effectively bridge the gap between insights and action. Their expertise ensures that businesses not only adopt the right tools but also implement them strategically for maximum impact.
Often, the most excellent value arises from combining both, leveraging generative AI vs predictive AI for content innovation alongside predictive insights from historical data. By selecting the right AI and predictive analytics tools, organizations can make more informed decisions, improve efficiency, and maintain a competitive edge.
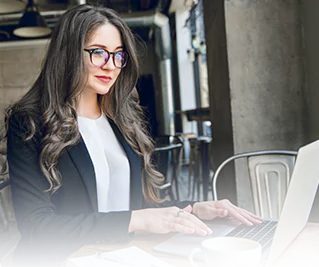